Innovative solutions for your success
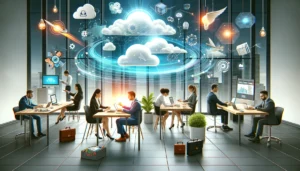
Showing the way through all the changes happening in technology and business, giving helpful tips and ideas to evolve and succeed in this digital world
AI business implementation is like adding a super-smart teammate to your company. These posts act as your guide, showing how to bring this smart tech on board, helping your business grow and thrive in the modern world.
Cybersecurity blog posts are your shield in the digital realm, offering strategies and tools to safeguard your online world. Empowering you with knowledge to protect against digital threats.
Showing the way through all the changes happening in technology and business, giving helpful tips and ideas to evolve and succeed in this digital world
AI business implementation is like adding a super-smart teammate to your company. These posts act as your guide, showing how to bring this smart tech on board, helping your business grow and thrive in the modern world.
Cybersecurity blog posts are your shield in the digital realm, offering strategies and tools to safeguard your online world. Empowering you with knowledge to protect against digital threats.
In the digital era, the data landscape has evolved exponentially, leading to a dire need for efficient data management and analysis. In the realm of Information Technology (IT), the emergence of data analytics has become indispensable for harnessing valuable insights from the vast pools of data generated daily. However, with the integration of Artificial Intelligence (AI) into data analytics, the paradigm has shifted dramatically, introducing a new era of advanced and actionable insights.
Data analytics is the process of examining datasets to draw conclusions about the information they contain. It involves various techniques and methods to interpret and analyze vast amounts of data to uncover patterns, correlations, and trends.
In the IT sector, data analytics plays a pivotal role in aiding businesses in making informed decisions. By analyzing data, IT professionals can identify areas for improvement, detect inefficiencies, and predict future trends, enabling organizations to stay ahead in an ever-evolving market.
The incorporation of AI in data analytics dates back to the early 2000s when rudimentary machine learning algorithms were used to process and interpret data. Companies like Acronis, a global leader in cyber protection, have integrated AI-driven data analytics into their backup and recovery solutions, enabling businesses to identify potential cyber threats and vulnerabilities in real-time.
AI has revolutionized data processing by automating tasks that were previously time-consuming and labor-intensive. Through the use of sophisticated algorithms, AI can quickly analyze massive datasets, extracting meaningful insights that aid in making informed business decisions. Zoho Analytics, a powerful self-service business intelligence and analytics platform, leverages AI to help businesses derive comprehensive insights from their data, empowering them to make data-driven decisions swiftly and efficiently.
One of the most significant contributions of AI to data analytics is its capability to facilitate predictive analysis. Companies such as Sophos, a global leader in next-generation cybersecurity, utilize AI-driven data analytics to predict and prevent potential security threats, enabling businesses to proactively safeguard their digital assets and sensitive information.
With the integration of AI into data analytics, ensuring the security and privacy of sensitive information has become a paramount concern. Safeguarding data from potential breaches and unauthorized access is essential to maintain the trust of customers and stakeholders.
Integrating AI into existing data analytics infrastructure can present several complexities, including compatibility issues, data migration challenges, and the need for substantial technological investments. Overcoming these hurdles requires meticulous planning and strategic implementation.
The successful implementation of AI in data analytics necessitates a workforce equipped with the necessary skills and expertise to operate and leverage AI-driven tools effectively. Training programs and educational initiatives are crucial in bridging the skill gap and empowering employees to embrace AI technologies.
AI-driven data analytics leverages machine learning algorithms to identify patterns and anomalies within datasets. These algorithms continuously learn from data, enabling businesses to make data-driven decisions and predictions based on real-time insights.
Natural Language Processing (NLP) is a branch of AI that enables computers to understand, interpret, and respond to human language. In data analytics, NLP facilitates the extraction of valuable information from unstructured data, such as customer reviews, social media posts, and survey responses, providing deeper insights into customer preferences and sentiments.
AI-powered image recognition technologies have transformed the way data is analyzed in various industries, including healthcare, retail, and manufacturing. By extracting information from images and videos, businesses can gain valuable insights into consumer behavior, product performance, and operational efficiencies.
The integration of AI-driven voice recognition technologies has streamlined operations within the IT sector. Voice-activated assistants and interactive voice response systems enable hands-free operation and efficient communication, enhancing productivity and user experience.
AI-driven data analytics empowers organizations to make informed decisions based on real-time insights and predictive analysis. By leveraging AI, businesses can identify market trends, consumer preferences, and potential risks, enabling them to make strategic decisions that drive growth and profitability.
The integration of AI into data analytics optimizes operational processes by automating repetitive tasks, reducing manual errors, and streamlining workflow management. This leads to improved productivity, cost-efficiency, and resource optimization, ultimately fostering a more agile and competitive business environment.
AI-driven data analytics enables businesses to deliver personalized customer experiences by understanding individual preferences, purchase behaviors, and engagement patterns. By analyzing customer data, businesses can tailor their products and services to meet specific customer needs, thereby enhancing customer satisfaction and loyalty.
Before integrating AI into data analytics, it is essential to establish clear objectives and goals that align with the organization’s overall strategic vision. Clearly defined objectives provide a roadmap for successful implementation and help measure the effectiveness of AI-driven data analytics.
Selecting the appropriate AI tools and platforms is crucial in ensuring the seamless integration of AI into existing data analytics processes. It is essential to evaluate the functionalities, scalability, and compatibility of AI solutions to meet the specific requirements and objectives of the business.
To adapt to the evolving data landscape, businesses must ensure that their AI-driven data analytics infrastructure is scalable and flexible. Scalability enables organizations to handle growing datasets and changing business demands, while flexibility allows for the seamless integration of new technologies and methodologies.
Maintaining data quality is critical for the accuracy and reliability of AI-driven data analytics. Regular data quality checks, data cleansing, and data validation processes are essential to ensure that the data used for analysis is accurate, complete, and up-to-date, leading to more reliable insights and informed decision-making.
The integration of AI in data analytics raises ethical concerns, primarily related to data privacy, transparency, and bias. It is imperative for businesses to establish ethical guidelines and practices to ensure that AI-driven data analytics remains unbiased, transparent, and aligned with legal and regulatory standards.
Maintaining the accuracy and reliability of data is a continuous challenge in AI-driven data analytics. Businesses must implement robust data validation and verification processes to detect and rectify any inconsistencies or errors in the data, ensuring that the insights derived from AI-driven analytics are trustworthy and credible.
While AI-driven data analytics offers numerous benefits, overreliance on AI can pose risks, including the loss of human judgment and critical thinking. Businesses must strike a balance between AI-driven insights and human expertise, fostering a collaborative approach that combines the power of AI with human intelligence.
The future of data analytics with AI is poised for continual innovation and advancements. Emerging technologies such as quantum computing, edge computing, and augmented analytics are expected to redefine the landscape of AI-driven data analytics, enabling businesses to extract deeper insights and unlock new opportunities for growth and innovation.
The integration of AI with the Internet of Things (IoT) and cloud computing is set to revolutionize data analytics by enabling real-time data processing, analysis, and decision-making. This convergence of technologies will facilitate the seamless exchange of data between connected devices, leading to more efficient and intelligent data-driven processes.
Several trends are shaping the future of AI-driven data analytics, including the democratization of AI, explainable AI, and AI-driven automation. These trends are expected to drive accessibility, transparency, and efficiency in AI-driven data analytics, making it more inclusive and user-friendly for businesses of all scales and industries.
AI integration has revolutionized data analytics in the IT sector, enabling streamlined processing and improved decision-making. Despite its benefits, addressing associated challenges and ensuring data quality is crucial for effective AI-driven data analytics. Looking ahead, the integration of AI with IoT and cloud computing is set to reshape the data analytics landscape, fostering further innovations.
Supercharge your data analytics with cutting-edge AI solutions! Sign up with PyramidBITS now and revolutionize your IT landscape.
We are dedicated to helping businesses like yours navigate the complex landscape of modern technology.
your dedicated IT partner